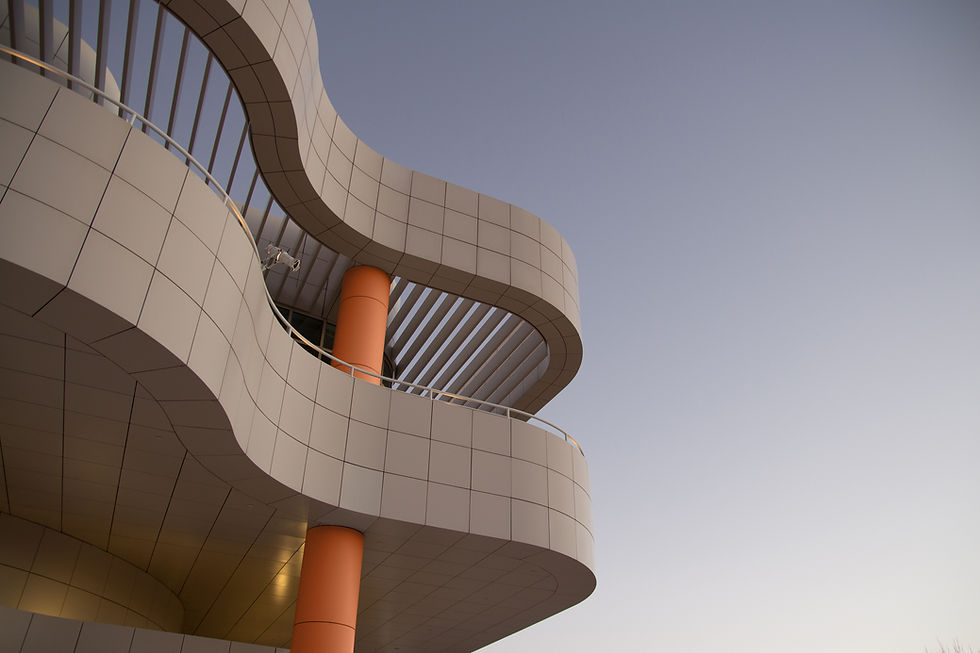
Revolutionizing Healthcare: How Predictive Analytics is Transforming Early Disease Detection
Jul 22
4 min read
0
3
0
The last couple of years have been completely different with respect to the face of healthcare due to the integration of advanced technologies into it. Of these, one outstanding factor for change has to be predictive analytics in health care, particularly in the early detection of diseases. This powerful tool, now powered by machine learning and artificial intelligence, is changing the way we think about preventive healthcare and management of diseases.
What is Predictive Analytics in Healthcare?
Predictive analytics in healthcare is the process of analyzing large amounts of medical data by applying statistical algorithms and machine learning techniques to come up with the predictive models for future health outcomes. In view of the reasons for early disease detection, data processing will be drawn from diverse sources, such as electronic health records, genetic information, lifestyle factors, environmental data, and wearable device data.
Integrating all these diverse data points, predictive models can project the chances of disease onset, hence facilitating its early interventions that might save countable lives.
Impact on Early Detection of Disease
Predictive analytics in the early detection of disease is a revolution for various reasons:
Improved Accuracy: The machine learning models are empowered to handle and analyze data at a scale and speed beyond the human clinician, hence leading to more accurate predictions.
Personalized Risk Assessment: Because a wide array of individual factors is taken into consideration, predictive analytics does indeed give a personalized risk profile, not like the old ways that were too generic to help.
Proactive Healthcare: Instead of reacting to symptoms, proactive measures can be taken by healthcare providers with predictive insights.
Cost-Effective Care: In most cases, early detection might assure more effective treatment and significantly reduce healthcare costs in the long run.
More broadly, predictive analytics can also be applied to identifying trends and risk factors across populations to inform larger public health strategies.
Applications in the Real World
Several such early applications of predictive analytics in disease detection are already promising, including:
Cardiovascular Disease: The risk of heart attacks and strokes is increasingly becoming accurate by models based on EHR data, lifestyle factors, and genetic information.
Cancer Detection: AI-empowered analysis of medical imaging data is enhancing the early detection of several cancers, including breast, lung, and skin cancer.
Diabetes Risk Assessment: Predictive models are able to identify people with a high propensity to develop type 2 diabetes and thus enable lifestyle interventions.
Mental Health: Analyzing data from social media activity or other behavioral data with predictive analytics can help in recognizing the first signs of mental health disorders.
Infectious Disease Outbreaks: Predictive models can project the extent of infectious diseases to take early containment measures.
Challenges and Ethical Issues
While predictive analytics is full of vast potential in healthcare, certain challenges need to be overcome, such as:
Data Privacy and Security: Sensitive health data must always be kept confidential and secure.
Bias in Algorithms: Predictive algorithms should not be biased towards one or another, leading to healthcare disparities.
Integration into clinical workflows: Successful implementation will require integration into current healthcare systems and processes.
Interpretability: For building trust and adoption by health providers and patients, predictive models should be interpretable and explainable.
Regulatory Compliance: Complex regulation surrounds health data and AI in healthcare, the navigation of which remains an ongoing challenge.
The Future of Predictive Analytics in Healthcare
Looking ahead to the future, we can find that the early detection of diseases continues to unfold new opportunities with predictive analytics. Some such emerging trends include:
Integration with IoT and Wearable Devices: Real-time data from wearable devices would contribute to the accuracy and timeliness of predictions.
Genomics and Personalized Medicine: genomic data should be integrated to approach a much more enhanced level of risk assessment and treatment plans.
Federated Learning: This would enable training predictive models across decentralized datasets, thus solving the privacy concerns and making use of larger datasets.
Explainable AI: Advancements in interpretable machine learning models increase trust and adoption among healthcare providers.
Conclusion
Predictive analytics for the early detection of diseases has really driven a paradigm shift in healthcare from reactive to proactive. It helps us identify diseases at an early stage through the use of data and advanced algorithms, intervene more effectively, and eventually save lives. With continuous fining of these technologies and overcoming the challenges involved, we are in a better position for healthcare compared to ever before.
It is a collective responsibility on the part of healthcare professionals, technologists, and policymakers to nurture this revolution so that the benefits accruing from predictive analytics are equitably and ethically derived across all segments of society.
As healthcare professionals, technologists, and policymakers, it's our collective responsibility to nurture this revolution, ensuring that the benefits of predictive analytics are realized equitably and ethically across all segments of society.
What are your thoughts on the role of predictive analytics in healthcare? How do you see it shaping the future of disease prevention and management? Share your insights in the comments below!
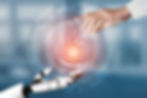